AI and ML in Finance: Facilitating Fraud Detection, Algorithmic Trading, And Personalization

4 Sep 2023
Igor Kelly

To succeed in the financial industry, you need well-founded data, flexibility, security, and a swift reaction time — all disciplines that computers are better at than people. Therefore, new data processing possibilities opened by artificial intelligence and machine learning in finance are having a considerable impact on the daily workflow.
The precise analysis, rapid processing capabilities, and predictive insights offered by AI and ML have not only elevated the efficiency of traditional practices. They also became indispensable tools in tackling the intricacies of financial fraud detection and refining algorithmic trading strategies.
In this article, we’ll discuss the significance of AI in banking and finance and which impact these technologies hold on the sector’s growth and stability.
AI in Fraud Detection
Across markets, fraudulent transactions cost 3.95 to 4.22 times the actual value lost. Businesses monitoring since pre-COVID (2019) saw a 10%-16% surge, with the pandemic compounding the financial fraud issue.
Fraud’s escalating complexity stems from the rapid digital transformation, amplified by the rise in mobile apps, digital wallets, and contactless payments that correlate with heightened fraud expenses. Amidst the added complexity of identity authentication, particularly during new account setup and logins, discernible patterns come to light:
- The rise of synthetic identities
- Inability to confirm the origination location
- A surge in the move to new digital
- Limited access to real-time risk assessment data and tools
- Increased volume of botnet attacks
AI-driven solutions became a lifeline for the financial industry, especially considering the factors above. Algorithms excel at adapting to evolving fraud patterns accelerated by digital transformation, enabling the identification of unusual behaviors across diverse transaction channels like mobile apps and contactless payments.
This adaptability empowers institutions to mitigate financial fraud risks by swiftly uncovering anomalies in the shifting consumer and merchant behaviors landscape. Below, we’ll examine multiple examples of how AI transforms the financial industry.
Algorithmic Trading and AI in Finance
AI is more than computer-aided trading. An AI is a fast-learning system that adapts to changing circumstances, evaluates thousands of factors in fractions of a second, puts them to each other, and derives the necessary action steps from them. Right now — after the end of the long phase of low-interest rates — stock market transactions are much more dependent on hard data and reliable calculations.
Therefore, predictive analytics is pivotal in informed decision-making within algorithmic trading. Here are examples of how AI in the finance industry works in practice.
Price movement prediction
Algorithmic traders use predictive analytics to analyze historical price data and identify patterns that precede certain price movements. For instance, if a specific stock’s price increases after a certain pattern occurs in the trading chart, the algorithm can trigger a buy order when similar patterns emerge again. This enables traders to make informed decisions based on the anticipated price movements.
News sentiment analysis
Predictive analytics also integrates news sentiment analysis to gauge market insights based on news articles, social media, and other sources. If the sentiment surrounding a particular stock becomes increasingly positive, the algorithm might predict an uptrend and adjust trading strategies accordingly.
Event-based trading
Predictive analytics can foresee the impact of specific events on market behavior. For example, suppose trading algorithms detect an upcoming earnings announcement for a company. In that case, it can predict how the stock’s price might move in response to the earnings report.
Using automated anomaly detection, traders can execute trades swiftly and capitalize on market opportunities more effectively.
Moreover, AI can unveil several hidden market trends that traders might not easily identify through traditional analysis methods, such as:
- Correlations across diverse data streams. AI can analyze social media sentiment, news events, and financial indicators to uncover correlations that impact stock prices. In 2017, the hedge fund Numerai used AI to find correlations between obscure data, like satellite images of parking lots and weather patterns, to predict stock market movements.
- Temporal patterns and seasonality. Analyzing historical data, AI can identify recurring trends that align with specific times of the day, week, month, or year. For instance, AI algorithms can help identify the “January Effect,” where stocks tend to rise at the beginning of the year.
- Public opinion. AI can analyze public sentiment from news articles, social media, and financial reports. For instance, during the GameStop trading frenzy in early 2021, AI algorithms helped track the sentiment around the Reddit-driven movement and its effect on stock prices.
- Geopolitical and macroeconomic influences. During Brexit negotiations, AI could analyze political speeches, economic reports, and trade data to forecast the potential effects on currency pairs and stock markets.
AI’s ability to process vast amounts of data, recognize patterns, and adapt to changing conditions empowers traders to discover impactful market trends. Furthermore, finance technology helps businesses make informed decisions that go beyond the scope of traditional analysis methods.
Want to break the bank with a mind-blowing trading app? Request a tailor-made solution from a software development company with experience applying AI in fintech domain.
Applying AI in FinTech for enhanced personalization
A recent study by Nvidia, a leading US provider of AI computing, asked 500 experts from the financial industry in North, Central, South America, and Europe about the most important AI trends. The result: 22% of companies surveyed noted fraud identification as one of the most important trends. Almost half of the survey participants expressed confidence that AI will increase their company’s annual revenue by at least 10%.
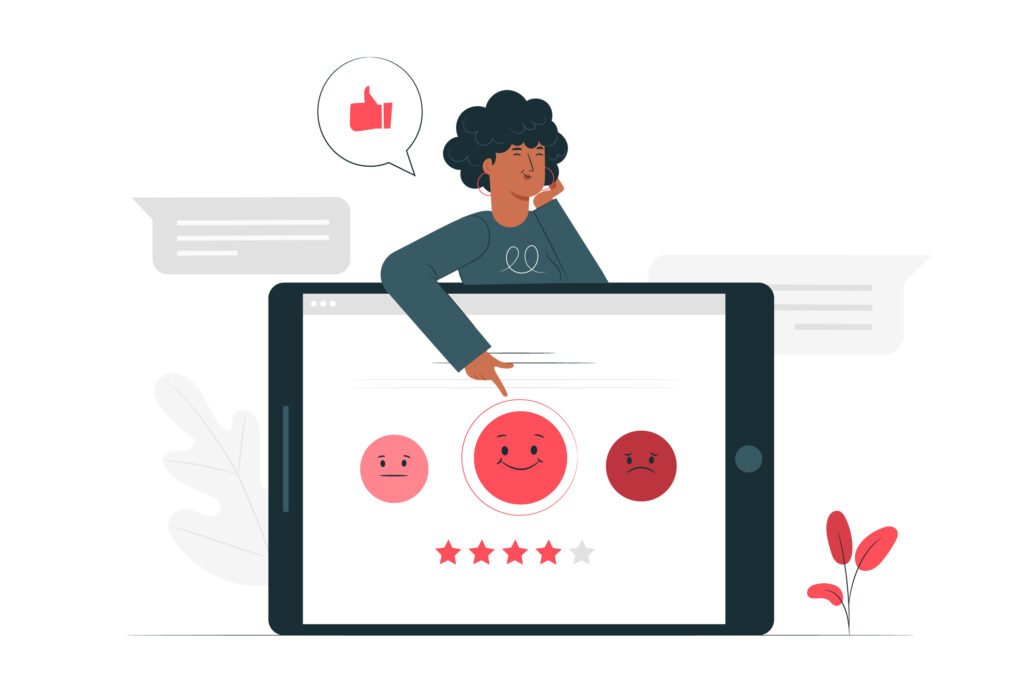
Even though 28% of the participants also state that the technologies are still error-prone and often lack meaningful data to train the AI models, there already are multiple positive use cases.
Let’s look at how companies in the financial sector have improved their personalization and customers’ experience by adopting AI/ML technology.
Chatbots for customer support
Financial institutions implement AI-powered chatbots to provide instant customer support and answer queries.
Capital One, for instance, uses a virtual assistant named “Eno” that helps customers with their account information, balances, and transaction history. Moreover, it even provides spending insights, thereby enhancing customer engagement and satisfaction.
Automated loan underwriting
Financial institutions leverage ML to automate and enhance the loan underwriting process.
ZestFinance, for instance, uses ML algorithms to analyze a broader range of variables, including alternative data sources such as rental history and utility payments, to assess credit risk more accurately. This enables lenders to extend credit to individuals who might have been overlooked by traditional credit scoring methods, fostering financial inclusion while maintaining responsible lending practices.
Personalized product recommendations
Machine learning in finance technologies analyzes customer transaction history and behavior to offer personalized product recommendations.
PayPal utilizes ML to suggest relevant financial services, such as loans or credit options, to individual users based on their transaction patterns, enabling more tailored offerings.
Credit scoring and loan approval
AI-based credit scoring models conduct narrow data analysis to evaluate a wider range of data sources. It helps to assess an individual’s creditworthiness more accurately.
LenddoEFL, a credit scoring company, employs ML to analyze non-traditional data, such as social media behavior and smartphone usage, to enable credit access for individuals lacking traditional credit histories.
Customized investment strategies
Advisory firms employ AI in finance operations to develop personalized investment strategies for clients based on their financial goals, risk tolerance, and market conditions.
Wealthfront, a robo-advisor platform, uses predictive modeling based on ML algorithms to craft diversified portfolios aligned with each client’s preferences and objectives.
Behavioral analysis for upselling
AI analyzes customer behavior to identify opportunities for upselling and cross-selling relevant financial products.
Bank of America’s virtual assistant, “Erica,” examines user transaction patterns to suggest potential savings or investment options, leading to improved financial decisions.
These use cases highlight how AI and machine learning in fintech inspire companies to personalize their offerings and streamline operations, ultimately improving customer satisfaction and loyalty.
Do you want to create a fintech project from scratch or take an existing one to the next level? Partner with a trustworthy fintech software development provider who develops software with a risk-aware culture in mind.
Challenges and Opportunities of AI in Fintech
Besides the numerous benefits, using AI in finance industry poses challenges, notably data protection and cybersecurity. We compiled the specific AI-associated risks:
- Complex deep learning verification. The intricacy of deep learning algorithms poses difficulty in result verification.
- Frequent recalibration. The synergy of self-learning algorithms and daily incoming data shortens recalibration cycles, blurring calibration-validation boundaries and hindering comprehensive quality control.
- Intense automation. Rapid scaling ensues as algorithms automate non-standardized processes and decisions, necessitating robust monitoring solutions integrated into banks’ IT structures.
- Risk of discrimination. Data collection and usage pose discrimination risks due to inadequate information about certain groups, leading to generalized decisions adversely affecting creditworthiness. A data governance strategy is imperative to regulate data handling for financial institutions.
The EU suggested using a regulatory compliance framework that categorizes risks of AI in banking and finance into four distinct levels:
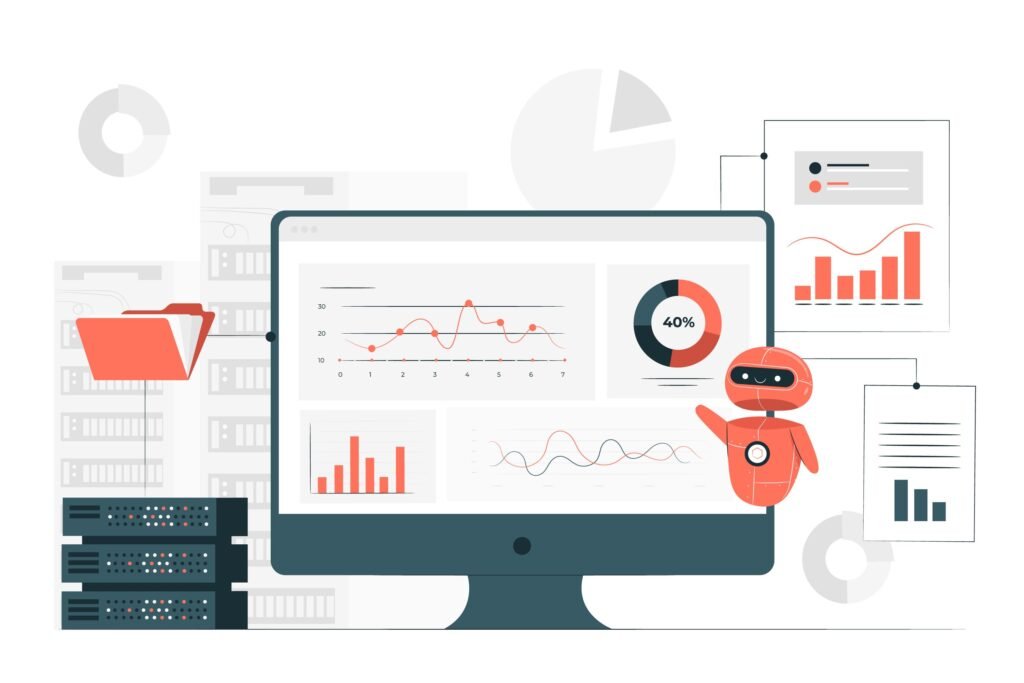
- Unacceptable risk, designating AI applications that pose substantial threats to safety, ethics, or legal compliance. Such risks are deemed unacceptable and warrant stringent regulatory intervention.
- High risk with AI applications carrying significant risks necessitating comprehensive risk management and regulatory oversight to minimize potential harms.
- Limited risk where applications require certain monitoring and controls to ensure responsible and safe deployment.
- Minimal or no risk use cases requiring only basic oversight to maintain their responsible implementation.
Pretend you’ve evaluated the risks properly. Now, how to address them in your company and comply with data privacy? Combat them considering the following framework conditions for AI.
Choose the right technology and build a well-founded enterprise architecture.
The success of data-driven AI applications relies on high-quality models, demanding dependable and current data. Robust data management is vital. Moreover, effective AI and data analytics hinge on contemporary tools, platforms, and infrastructure. Finally, integrating these elements strategically within the enterprise architecture ensures purposeful implementation.
Properly align the business model with AI
The use of machine learning in finance has long since become a strategic issue. Any disruption as an external factor affects the business model and has a lasting effect. Therefore, the connection between technology and business model no longer only means making something known better or more efficient but goes beyond that — and it’s important to remember when adopting new technologies.
Leverage operating model and governance for AI
The operating model faces the challenge of delivering business-defined products and services to customers, necessitating adjustments in people, processes, organization, and leadership for data-driven technologies. Incorporating roles like data analysts, engineers, and scientists demands strategic organizational integration.
Ultimately, success in AI implementation hinges on identifying valuable use cases and establishing supportive conditions regarding financial security. On the part of the supervisory authorities, using AI-based tools will also be viewed critically.
Conclusion
By harnessing the power of advanced data analytics and pattern recognition, AI and ML technologies have remarkably enhanced fraud prevention accuracy, optimized trading strategies, and delivered personalized financial services to customers.
Looking ahead, the future of artificial intelligence and machine learning in finance appears promising, poised to revolutionize manual tasks such as risk assessment and transaction validation through automated processes. Furthermore, these technologies will increasingly streamline complex analytical tasks, facilitating real-time decision-making and predictive insights.
By embracing these technologies responsibly and nurturing a collaborative ecosystem, financial institutions can safeguard their operations and pave the way for a future where enhanced customer experience and ethical considerations harmoniously drive progress, resilience, and success.
If you want to use artificial intelligence’s enormous potential and develop projects in a value-adding manner, contact Lightpoint’s expert today for a personalized consultation.