How Does Big Data Contribute to FinTech Success?

6 Feb 2024
Ilya Lashch
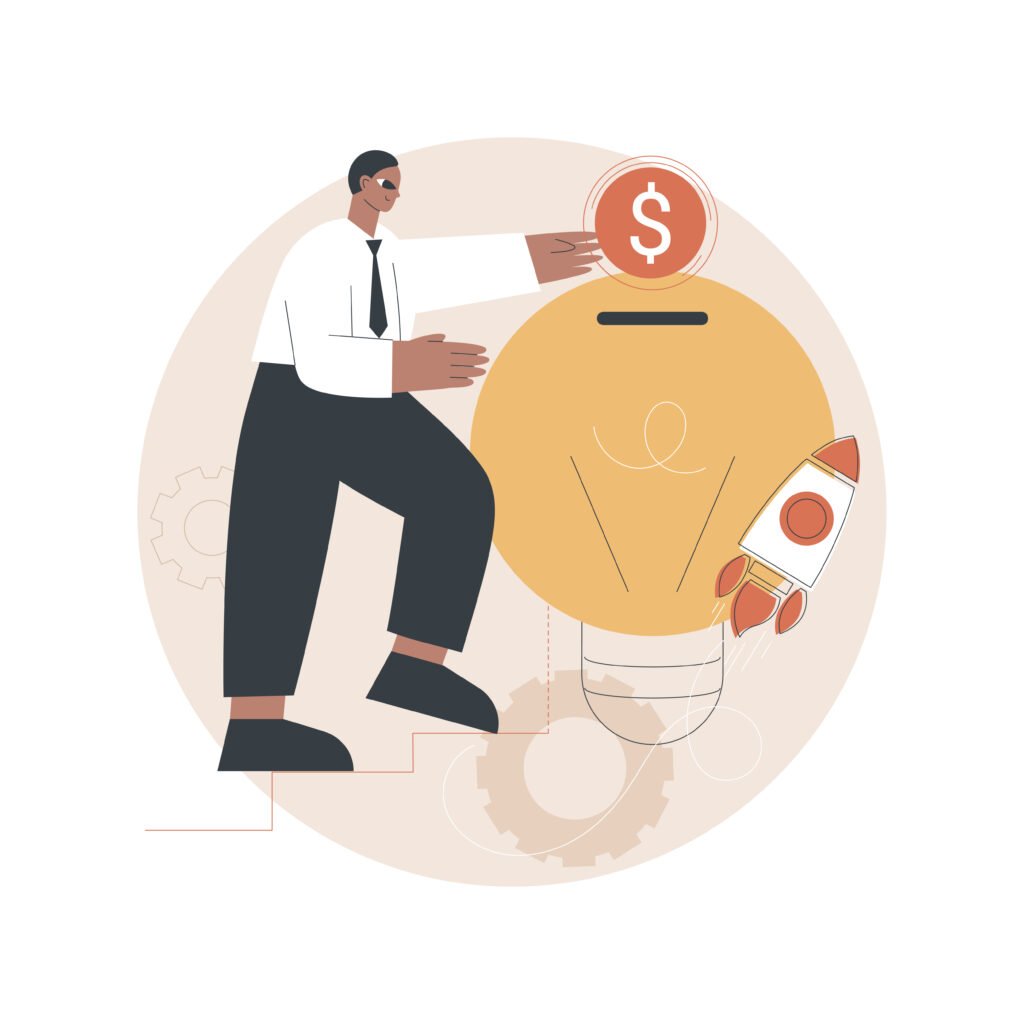
Currently, around 20,000 FinTechs worldwide are ambitious, act dynamically, and know that with AI, machine learning (ML), and big data solutions they can set themselves apart from the competition, especially conventional financial institutions.
In short, big data in FinTech refers to the large volume of structured and unstructured financial data generated from various sources, including transactions, market trends, customer behavior, and regulatory filings. This data is analyzed using advanced analytics tools to uncover valuable insights, enhance decision-making processes, and drive innovation within the financial industry.
What is big data impact on financial processes? We’ll provide an answer in this article, explore the main benefits and pitfalls, and explore the most promising big data use cases in FinTech.
The Synergy of FinTech and Big Data
Big data in finance industry encompasses a suite of software tools designed to manage, collect, analyze, organize, and access structured and unstructured data effectively. The global big data technology market, valued at $309.43 billion in 2022, is projected to witness substantial growth, reaching $842.17 billion by 2030, with an average annual growth rate of 13.6% during the forecast period, according to Forrester research.
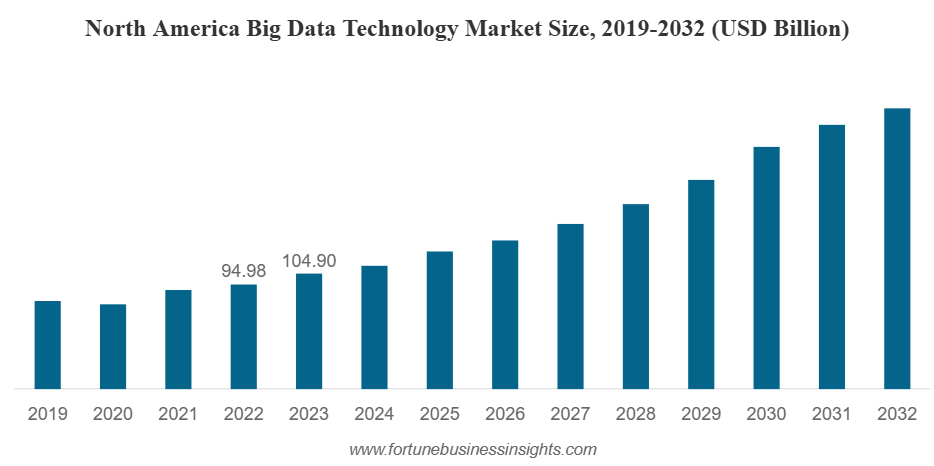
The significance of big data technology is underscored by increasing investments and spending on technology projects by both government and private entities. According to the Worldwide Big Data and Analytics Spending Guide (2022), spending on big data technology is expected to surge 1.6 times by 2025. Moreover, the expanding internet penetration and the widespread adoption of cybersecurity, the Internet of Things (IoT), 5G infrastructures, and machine learning in financial technology are poised to fuel market growth.
Here are the key drivers of big data in the financial industry:
- Enhanced decision-making: Big data analytics in finance empower companies to make data-driven decisions, enabling more accurate risk assessments, personalized offerings, and improved operational efficiencies.
- Fraud detection and prevention: Real-time data processing in financial services helps detect and prevent fraudulent activities, safeguarding financial institutions and their customers from financial losses and reputational damage.
- Customer insights and personalization: Big data technology enables financial institutions to gain deeper insights into customer behavior, preferences, and trends, facilitating personalized product recommendations, targeted marketing campaigns, and enhanced customer experiences.
Big data in FinTech changes how companies operate. Let’s look at examples of how FinTech ventures use big data to make payments safer, assess credit, prevent fraud, and offer personalized financial services.
- 1. Stripe. A leading online payment processing platform, utilizes predictive analytics in finance to analyze transaction patterns and detect real-time fraudulent activities. By leveraging machine learning algorithms, Stripe enhances security measures and minimizes risks for merchants and consumers, ensuring seamless and secure payment transactions.
- 2, Adyen. A global payment technology company, harnesses big data analytics to provide insights into customer payment behaviors and preferences. Real-time data processing in financial services for merchants helps Adyen optimize payment processes, improve conversion rates, and enhance overall customer experiences across multiple channels.
- 3. Kabbage. An online lending platform for small businesses, leverages big data analytics to assess creditworthiness and make data-driven lending decisions. By analyzing a wide range of financial and non-financial data, including transaction history, social media activity, and business performance metrics, Kabbage provides quick and efficient access to capital for small businesses, enabling them to grow and thrive.
- 4. Plaid. A financial technology company specializing in data connectivity, utilizes big data analytics to aggregate and analyze financial data from various sources. By providing secure access to bank account information and transaction data, Plaid enables developers and financial institutions to build innovative financial products and services, ranging from budgeting apps to investment platforms, that empower consumers to manage their finances more effectively.
Ready to harness the power of big data in finance industry? Our comprehensive big data development services unlock new opportunities and propel your business forward.
Benefits of Big Data for FinTech
This convergence has garnered interest from Big Tech companies, aiming to leverage payment transactions as a gateway to access and analyze the amalgamation of personal and financial data. As this bridge expands, it promises transformative insights and potential advancements in credit assessment methodologies.
Below, we list the benefits of utilizing big data in FinTech:
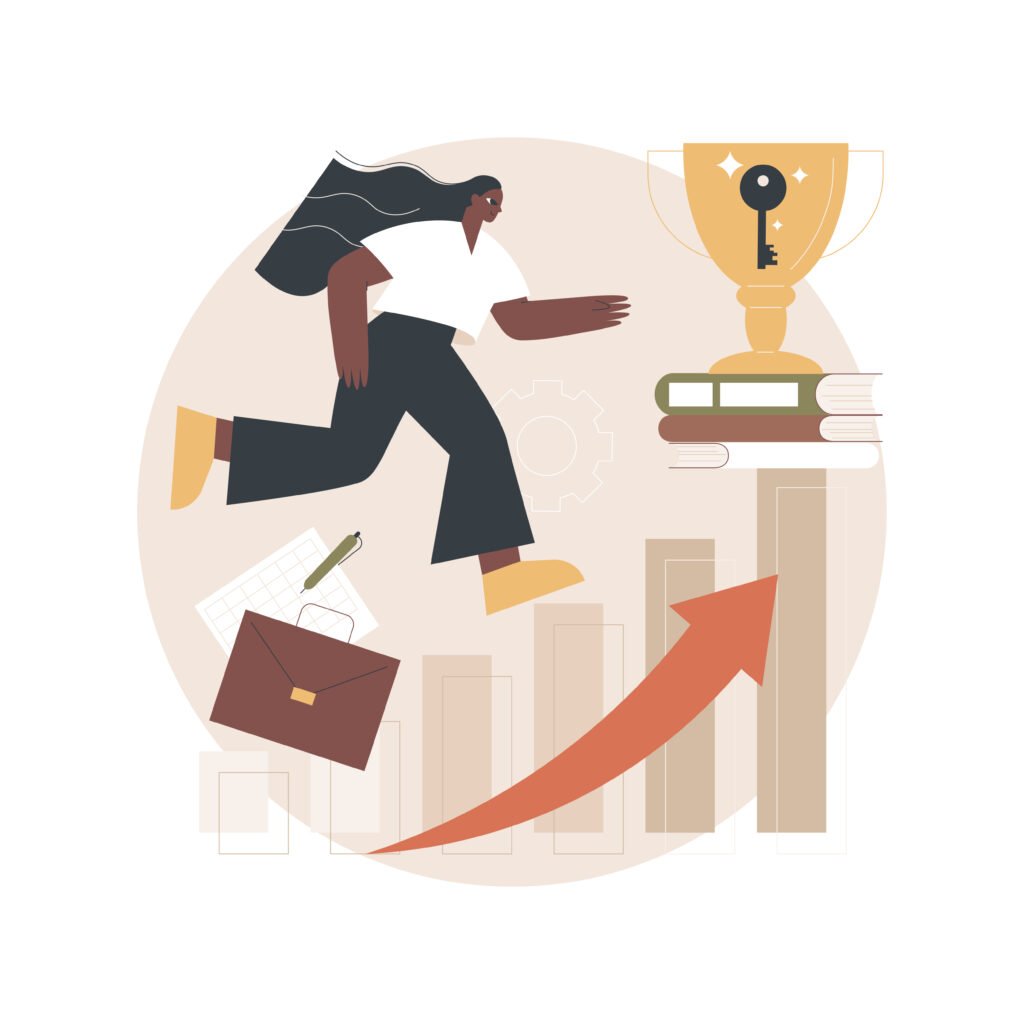
1. Improved customer segmentation: Big data allows financial institutions to segment customers more effectively based on various parameters such as demographics, behavior, and transaction history. This enables targeted marketing efforts and personalized product recommendations tailored to specific customer segments.
For instance, a bank may use big data analytics to identify and target high-net-worth individuals with personalized investment opportunities by analyzing their transaction history, investment preferences, and asset portfolio.
2. Customer-focused services: By leveraging big data analytics, financial institutions can gain deeper insights into customer preferences, needs, and behaviors. Data insights for financial services enable the development of customer-focused services and experiences that address specific pain points and deliver added value to customers.
For example, a credit card company might analyze transaction data to offer customized rewards and benefits tailored to individual spending habits.
3. Improved security protocols: Given the predicted annual 19% CAGR growth of the fraud detection and prevention market, this topic draws much attention. Big data analytics enhance cybersecurity protocols by analyzing vast amounts of transaction data in real time to identify suspicious patterns and anomalies.
Such a proactive approach helps financial institutions mitigate risks and protect customer assets from fraud. For instance, a payment processing company may use machine learning algorithms to detect and block fraudulent transactions before they occur, safeguarding both merchants and consumers.
4. More streamlined operations: FinTech data strategy implies automating manual processes, optimizing workflows, and reducing operational inefficiencies. By analyzing data from various sources, organizations can identify areas for improvement and implement measures to enhance operational efficiency and productivity.
For example, a retail bank may use big data analytics to automate loan approval processes, reducing turnaround times and improving customer satisfaction.
5. More accurate credit risk assessment: Intelligent tools provide enhanced risk management through data into credit risk assessment by analyzing various data points, including credit history, income levels, and repayment behavior. This enables lenders to make informed decisions about extending credit to borrowers and setting appropriate interest rates.
For instance, a peer-to-peer lending platform may use machine learning algorithms to assess credit risk and determine loan eligibility for borrowers based on their financial profiles.
The industry knows multiple examples of how big data help FinTech businesses. However, there are some undeniable disadvantages.
Challenges and Barriers in Big Data for FinTech
For financial providers, leveraging cutting-edge technologies presents both immense opportunities and formidable challenges. From ensuring data accuracy and completeness to navigating complex regulatory frameworks and security concerns, businesses face multifaceted challenges that require strategic solutions for successful integration and utilization of big data. Let’s look at three of them in more detail:
1. Data quality concerns: Ensuring data accuracy, completeness, and reliability remains a significant challenge for FinTechs. Unclean or incomplete data can distort big data analytics outcomes, leading to incorrect conclusions and hindering the desired transformation results.
Utilizing data quality management platforms can assist in streamlining data cleansing processes, enhancing data integrity, and maintaining high standards of accuracy and completeness across their datasets.
2. Integration of unstructured data: Merging unstructured data, such as videos, emails, and social media posts, with structured internal data presents a technical and organizational challenge for FinTechs. Developing the right approach to integrate and analyze unstructured data alongside traditional structured data sources requires substantial effort and expertise.
Deploying sophisticated data integration and analytics software solutions, such as Apache Nifi or IBM Watson, enables businesses to effectively manage and analyze unstructured data like videos, emails, and social media posts alongside structured data sources. These platforms leverage machine learning and natural language processing capabilities to streamline the integration process and derive actionable insights for informed decision-making.
3. Limited experience with external data: FinTech data management primarily focuses on utilizing big data analytics for internal data rather than incorporating external data sources like social media due to limited experience and expertise in handling such data. Improving the quality and reliability of external data for algorithmic evaluation poses additional challenges, yet it is essential for developing comprehensive analytical applications and deriving meaningful insights.
Specialized data integration platforms usually streamline the incorporation of external data sources, while also providing tools for data cleansing, validation, and algorithmic evaluation. These solutions enable fintech businesses to enhance the quality and reliability of external data, facilitating the development of comprehensive analytical applications and the derivation of meaningful insights.
4. Data security: One of the biggest challenges in big data lies in data protection and security, as financial data is highly sensitive. Responsible handling and protection following statutory data protection regulations and the Federal Financial Supervisory Authority (BaFin) requirements is essential for FinTechs and banks and requires special investments in cybersecurity.
Software solutions such as encryption software, intrusion detection systems, and access control mechanisms help maintain data security by encrypting sensitive information, monitoring network activities for suspicious behavior, and regulating access to data based on user privileges, thereby safeguarding financial data from unauthorized access and breaches.
Lightpoint’s specialists compiled a list of tips for ensuring cybersecurity when utilizing big data tools in the financial industry:
- Mindful information sharing: Carefully consider the data you provide to financial service providers and be cautious about sharing sensitive information online or on social networks.
- Review privacy settings: Regularly review and adjust privacy and data protection settings for apps and digital services, ensuring that the security measures align with your needs and preferences.
- Read data protection policies: Thoroughly read and understand the data protection information before consenting to data processing. Don’t hesitate to ask the relevant service provider for clarification if clarification is needed.
- Exercise informed consent: Only consent to process your data if you fully agree with how the service provider intends to use it. Be proactive in understanding and agreeing to the terms of data usage.
- Report violations promptly: If you believe your personal rights are infringed upon, file a complaint with the service provider’s support team. If the resolution isn’t satisfactory, escalate your concerns to the appropriate regional data protection authority for further assistance.
Business leaders should consider partnering with outside companies or experts to expand their knowledge and experience in big data and analytics. External partners can help introduce new data security technologies and ensure a profitable big data impact on financial processes.
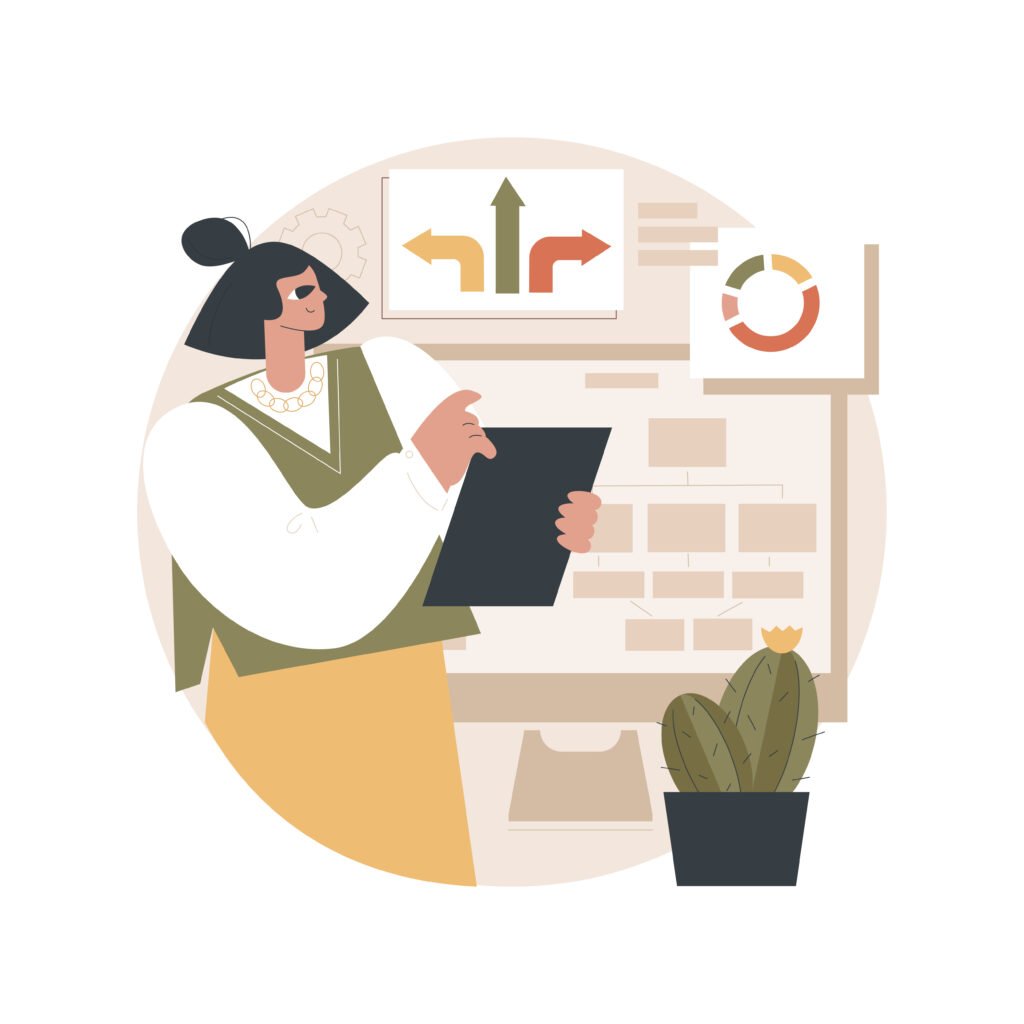
Choosing the Strategy for Big Data Integration in FinTech
Today, we all want to use financial products and services that are fluid, user-friendly, quickly available, and cost-effective. And we expect to build relationships with our bank that are rewarding and emotionally appealing to us. To achieve a higher quality customer experience, adopting advanced analytics in financial technology requires a solid understanding of the implementation process.
You can leverage the following tips for developing a successful advanced analytics strategy and improving financial decision-making with big data:
- Stay open to new solutions: Banks and financial institutions must embrace new approaches offered by big data analytics, recognizing that valuable insights often lie beyond conventional data sources. Utilizing analytics enables more precise answers to economically relevant questions, driving innovation and competitive advantage.
- Prioritize use cases: Identifying and prioritizing use cases is crucial to successfully integrate analytics into regular banking processes. FinTechs can avoid the pitfalls of failed pilot projects and effectively scale initiatives by focusing on areas where analytics can deliver measurable results, such as customer loyalty programs and dynamic pricing.
- Adhere to the long-term vision: Decision-makers should maintain a long-term perspective when implementing analytics solutions, considering how each use case contributes to the bank’s overall strategic objectives. By aligning analytics initiatives with long-term goals, banks can maximize the value derived from their investment in big data technologies.
By following these recommendations, business leaders can ensure their organization reaps the benefits of big data and analytics and achieves a competitive advantage. Lightpoint experts can assist you in building a data-based ecosystem of any complexity with custom FinTech software development services. Whatever FinTech data applications you want to create, we can provide the right expertise.
Final Thoughts on Big Data in FinTech
In conclusion, the effective utilization of big data in FinTech hinges upon a modern data management framework capable of integrating and normalizing data from diverse external and internal sources. By adopting such a concept, FinTech businesses can unlock the full potential of data analytics, enabling informed decision-making and driving innovation across all departments.
A surge in data volumes sourced from diverse channels and third-party providers requires innovative data-backed software solutions to handle them. Lightpoint experts would happily provide a consultation and deliver a custom big data solution to empower your financial services.