Strategic Selection: Choosing the Right Data Capture Technique for Your Business

25 Jun 2024
Ilya Lashch
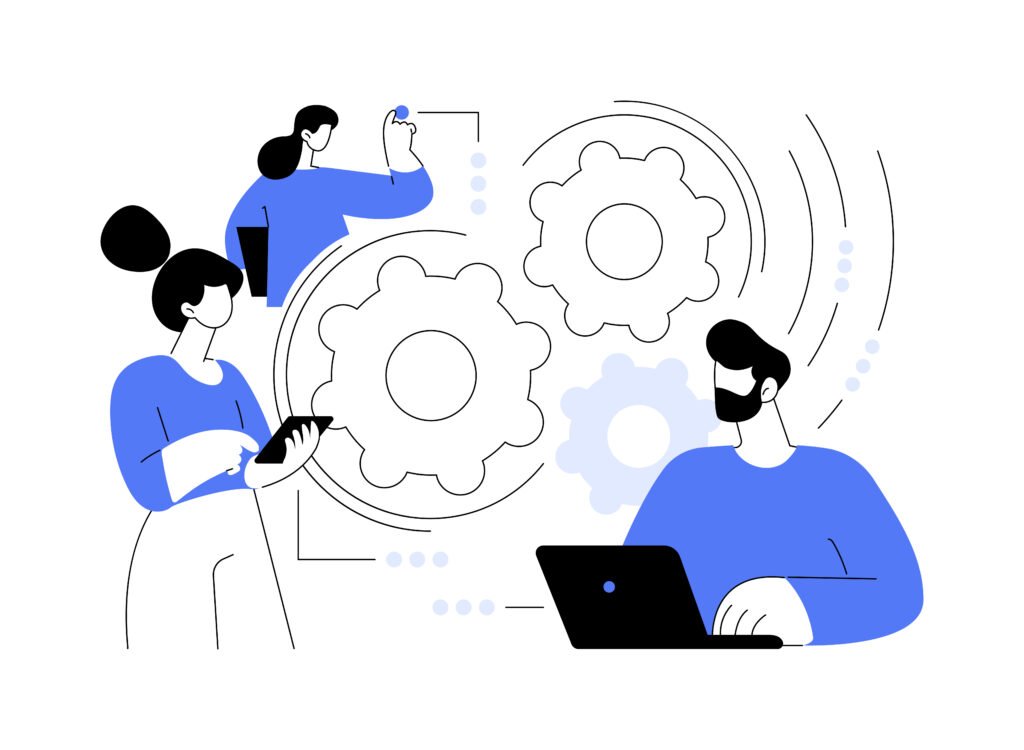
Summary
In the article, we dive into the intricacies of data capture processes, covering topics from structured to unstructured data, the article explores various data capture techniques and their applications in business settings. The article also covers how businesses can leverage effective data capture strategies to drive informed decision-making, personalize customer experiences, enhance automation, and more.
Data Capture Process Explained
Data collection is crucial to the functioning of companies. With 120 zettabytes of data being created (globally) in 2023 alone, it’s no surprise that data is considered one of the most valuable assets for businesses. So, what is data capture? In short, its process looks like this:
- The data is received as an image, email, document, etc.
- Data is extracted and collected (manually or automatically) to be stored in a digital format.
- The captured data can then be used for further processes.
Data capture techniques are used in many areas, such as research, business, or public administration. It is the process of obtaining and measuring information about variable interests systematically that enables information retrieval and objective assessment of specific topics under investigation.
Lorem ipsum dolor sit amet
Lorem ipsum dolor sit amet
Generally, two types of business data capture can be distinguished: primary and secondary.
- Primary data is obtained directly from the source, for example, via surveys, interviews, experiments, or observations.
- Secondary data is existing data collected by others.
Both have their value and applications in different contexts. It is also important to emphasize that qualitative or quantitative data can be collected.
- Qualitative data is typically text-based and more descriptive, while quantitative data is numerical and measurement-based. Qualitative data provides deep, detailed, and contextual information. This type of data collection can explore the “why” and “how” of a given topic or context.
- Quantitative data collection allows far-reaching and representative results to be achieved and hypotheses to be tested. The focus here is primarily on numbers, statistics, and the measurement of variables.
Finally, it should be mentioned that choosing the best fit from different data capture methods depends heavily on the type of information desired, the available budget, the time span, and the available resources. With the right data harvesting method, high-quality data can be collected, leading to informed decisions, predictions, and insights.
Data transformation from silos to organized databases offers companies numerous advantages. For example, at a high level, it allows a company to make better decisions that optimize marketing campaigns, develop superior products, and improve customer service.
Here are the key benefits of collecting data:
- Efficient data process: Data ingestion facilitates the seamless gathering of customer feedback from multiple channels such as social media, email, and surveys, enabling businesses to analyze sentiments and identify trends to enhance product development and customer satisfaction strategies.
- Data centralization: Business intelligence tools centralize data in a single repository or system. Data aggregation makes it easier to manage and use. For example, worldwide enterprises use AI software development solutions to consolidate sales data from their regional offices. Customized centralized repositories allow executives to receive timely insights from real-time data capture and make data-driven decisions more efficiently.
- Integration with analytics tools: The data acquisition process can seamlessly integrate various analytics and visualization tools for advanced analytics, reporting, and business intelligence. Popular analytics tools like Tableau and Power BI allow businesses to integrate collected data for advanced analytics and visualization. For instance, Tableau enables users to create interactive dashboards and reports from diverse data sources, aiding in uncovering trends and patterns. Similarly, Power BI offers robust data modeling capabilities, allowing users to transform raw data into meaningful insights through customizable reports and visualizations, empowering informed decision-making across the organization.
- Operational efficiency: Automating digital data collection with advanced tools like object recognition software reduces manual effort and improves operational efficiency, freeing resources for more strategic tasks. Many retail chains implement automated data capture in their inventory management system. Companies can automatically capture data about each product’s movement in real time by utilizing barcode scanners at the point of sale and RFID readers in their warehouses. The automated data capture process reduces the need for manual data digitization, minimizes errors, and provides accurate insights into inventory levels.
Types of Data Captured
All data for collection are in different formats and fall into two main categories: structured and unstructured. The third one, semi-structured data, comprises two types.
1. Structured data
Structured data is data structured in a predetermined format before being stored in data storage. This is often referred to as schema-on-write. The best example of structured data is the relational database: the data is formatted into precise fields, such as credit card numbers or addresses so that it can be easily queried using SQL.
Structured data has three main advantages:
- Easily usable by machine learning algorithms: The biggest advantage of structured data is that machine learning can easily use it. Because structured data is organized, it can be easily edited and queried.
- Easily usable by business users: Another advantage of structured data is that an average business user knowledgeable about the topic the data relates to can also use it. You don’t need a deep understanding of the different types of data or the relationships between the data. Structured data mining enables business users to access self-service.
- Better access to more tools: Structured data also has the advantage of being used for a much longer time, as it was the only option in the past. This means more data input methods for analyzing structured data have already been tried and tested. Data managers have a wider range of products when working with structured data.
The disadvantages of structured data are primarily related to the lack of flexibility. Here are some potential disadvantages of structured data:
- A predefined purpose limits the possible uses: Defining data in schema-on-write is one of the biggest advantages of structured data. However, it is also true that data with a predefined structure can only be used for its intended purpose. This limits the flexibility and possible applications.
- Limited storage options: Structured data is typically stored in data warehouses. Data warehouses are data storage systems that follow rigid schemas. Any change in requirements means that all structured data must be updated to meet the new requirements. This costs time and resources. Some costs can be saved by relying on a cloud-based data warehouse, which offers greater scalability and eliminates maintenance costs associated with operating devices on-premises.
2. Unstructured data
Unstructured data is data that is stored in a native format and is not manipulated until it is used. This is also called schema-on-read. Unstructured data also has strengths and weaknesses depending on business needs. Benefits include:
- Freedom of native format: Because unstructured data is stored in its native format, the data is not defined until it is needed. This leads to a larger pool of possible applications because the purpose of the data is customizable. Data scientists only have to prepare and analyze the data that is needed. Saving in native format also allows for a wider range of file formats in the database because data cannot only be saved in a specific format. What this means for the company is that it has more data to draw from.
- Faster data accumulation: Another advantage of unstructured data is that data can be accumulated faster. Since the data is not predefined, it can be captured quickly and easily.
- Data lake storage: Unstructured data is often stored in data lakes in the cloud, which provide massive storage capacity. Cloud data lakes also enable pay-as-you-use pricing concepts for storage. This helps reduce costs and scale easily.
However, the use of unstructured data comes with the following disadvantages:
- Data science know-how required: The biggest disadvantage of unstructured data is that you need data science know-how to prepare and analyze the data. An average business user cannot use unstructured data as it is because it is undefined/unformatted. If you want to use unstructured data, you have to know the topic or subject area of the data and how to put the data into context to use it.
- Special tools: Besides know-how, unstructured data also requires special processing tools and data enrichment techniques. This leaves a data manager with a limited selection of products for unstructured data, some of which are still in their infancy.
Unfortunately, over 80% of business data is stored in unstructured formats such as documents, emails, PDF files, and images. Transforming unstructured data into structured, searchable, and machine-readable data is considered one of the biggest challenges for companies. This process is necessary to use the data for a specific task or process, such as entering them into a computer program or using them in an Excel spreadsheet.
3. Semi-structured data
Semi-structured data lies between structured data and unstructured data. For example, video storage could contain structured data tags for each file, such as date, location, and subject. Metadata on multimedia files means that they are inherently semi-structured data. Due to the mix of structured data and unstructured data types, the data is semi-structured. Using semi-structured data instead of raw unstructured data can make analyzing the underlying unstructured data faster and easier.

Let’s examine the methods of data collection and a few data capture examples.
Traditional Vs. Modern Data Capture Methods
The type of data capture techniques used for both structured and unstructured data depends on the type of data storage. It can be one of the following:
- Relational databases
- NoSQL databases
- Data lakes
- Data warehouses and other
The traditional data capture methods include:
- Manual data entry: Inputting data by hand into digital systems or databases.
- Paper-based forms: Recording information on physical documents that are later manually transcribed or scanned.
- Barcodes and QR codes: Utilizing encoded symbols to represent data that specialized equipment can scan and interpret.
However, businesses increasingly favor modern data capture methods for efficiency, accuracy, scalability, and seamless integration with existing systems. These methods automate data collection, reducing manual effort and errors while ensuring reliable data outcomes. Additionally, they enable businesses to handle large datasets more efficiently with cutting-edge data management strategies, facilitating better decision-making. The most widely used methods include:
- Optical Character Recognition (OCR): The optical character recognition software converts scanned documents or text images into editable and searchable data. It enables businesses to digitize and extract information from physical documents, such as invoices or forms, for efficient data entry and analysis.
- Intelligent Character Recognition (ICR): ICR is a variant of OCR and is often used by banks and financial institutions. This technology can read handwritten characters and convert them into meaningful machine-readable data.
- Intelligent Document Processing (IDP): Using AI-based technologies combined with OCR, IDP can automate document processing workflows. IDP helps data scientists to extract and verify classified data.
- Automatic identification and data capture (AIDC): AIDC systems, including barcode scanners and RFID readers, automatically capture data from encoded symbols or tags attached to items or documents. Sensor technology streamlines inventory management and logistics processes by accurately identifying and tracking products throughout the supply chain.
- Mobile data capture: Mobile data capture refers to using smartphones or tablets to collect and input data directly into digital systems. Data entry automation enhances field data collection, enabling remote workers to capture information on the go, such as completing surveys, conducting inspections, or processing orders in real time.
- Internet of Things (IoT) sensors: IoT sensors gather data from connected devices embedded in physical objects or environments. These sensors collect various data types, such as temperature, humidity, or motion, and transmit them wirelessly to centralized systems for analysis, thus streamlining data capture. IoT sensor data is instrumental in predictive maintenance, environmental monitoring, and smart home automation applications.
To better describe how these data processing techniques work, let’s examine a few case studies illustrating their implementation.
1. Retail inventory management with RFID
Macy’s, one of the largest department store chains in the United States, implemented RFID technology in their stores to improve inventory accuracy and streamline operations.
By tagging merchandise with RFID tags, Macy’s could track inventory in real-time, reducing out-of-stock situations and minimizing excess inventory. Data capture technology significantly increased inventory accuracy, which improved customer satisfaction by ensuring products were available when needed.
2. Mobile data capture in field service
British Gas, a leading energy and home services provider in the UK, adopted mobile data capture solutions for their field service technicians.
Equipping technicians with mobile devices allowed them to access and update customer information, service histories, and job details in real time. This implementation improved efficiency by eliminating paperwork, reducing administrative overhead, and enabling faster response to customer inquiries and service requests.
3. OCR for document processing in healthcare
Mount Sinai Hospital in New York City implemented OCR technology to streamline the processing of medical records and forms.
By digitizing and extracting data from handwritten medical notes and forms, Mount Sinai Hospital improved the accuracy and speed of patient record management. Implementing data capture techniques reduced manual data entry errors, enhanced compliance with regulatory requirements, and enabled healthcare professionals to access patient information more efficiently, ultimately improving patient care outcomes.
Data Protection and Ethical Considerations
When conducting data extraction and collection processes, adhering to data protection guidelines and making ethical considerations is essential. These factors ensure the security, privacy, and trust of the individuals and institutions concerned. But what does that mean specifically?
Data protection is a fundamental requirement in all available data capture techniques. Personal data protection typically includes measures to protect user personal data, the confidentiality of the information collected, and how this data is stored and further processed.
Ethical considerations in data collection often relate to the handling of sensitive information, the transparency of the data capture technology, and, ultimately, respect for the individual rights of respondents. Ethics committees or advisory boards are often there to ensure these principles are adhered to.
Concerning data protection, the following points are important, for example:
- Data security officers in companies and organizations
- Training and education for employees
- Technical security measures such as data encryption
- The usage of reliable data recording techniques, regular reviews and audits
Ethical considerations can be ensured through the following measures:
- Advance consent forms
- Transparent information about the purpose of data collection
- Secure storage and use of data
- Possibility to revoke consent at any time
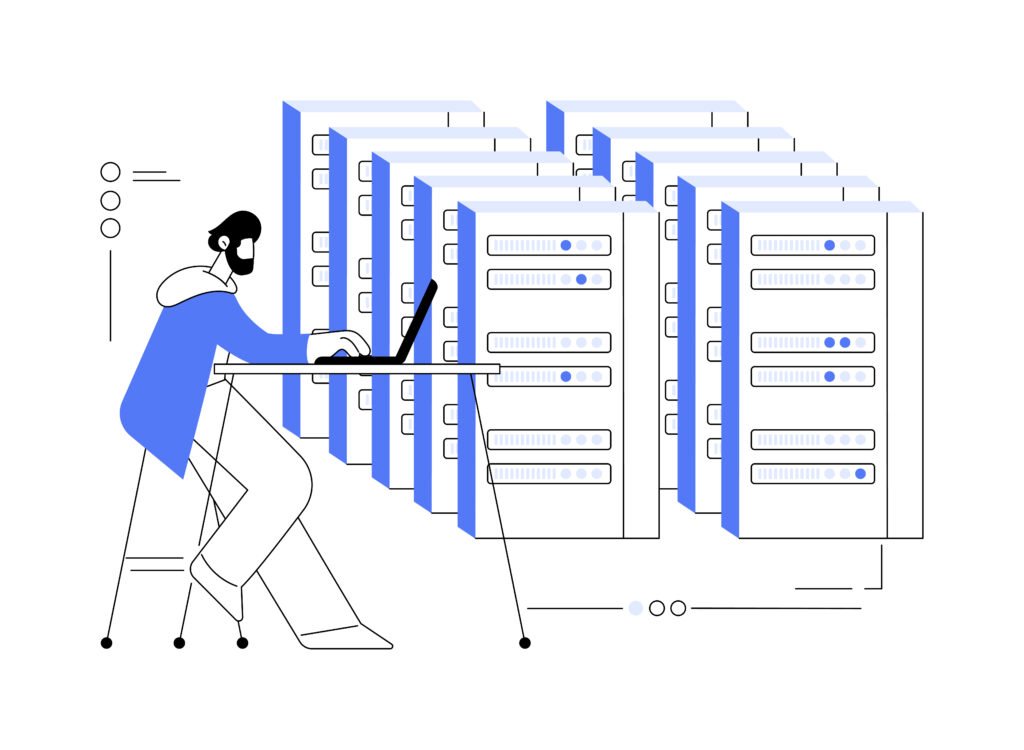
Overall, data protection and ethical considerations are essential parts of any data collection process. They create trust between those collecting data and those surveyed and ensure that the quality and integrity of the data obtained is maintained.
Best Practices for Effective Data Capture
As an experienced tech provider specializing in data collection, we adhere to the following best practices to ensure effective data capture:
1. Data quality assurance:
- We employ validation rules to ensure that the data captured meets predefined criteria, such as format, range, and completeness.
- Conduct regular audits and employ data verification processes to identify and rectify inconsistencies or inaccuracies in the captured data.
- Robust error handling mechanisms help to detect and correct errors during data capture processes, ensuring high data quality.
2. Standardization and normalization:
- We use standardized data schemas and structures to ensure consistency and uniformity in captured data across different sources and systems.
- Employ data cleansing methods such as deduplication, formatting, and standardization to normalize captured data and eliminate discrepancies.
- Industry standards and best data capture, storage, and processing practices facilitate interoperability and data exchange with external systems.
3. Security and compliance considerations:
- Lightpoint’s data security experts implement encryption protocols to safeguard sensitive data during transmission and choose trustworthy data storage solutions.
- We enforce role-based access controls that restrict access to sensitive data based on users’ roles and permissions, minimizing the risk of unauthorized access.
- Compliance with relevant data protection regulations such as GDPR, HIPAA, or PCI DSS is obligatory. Thus, we implement measures to protect personal and sensitive data and maintain audit trails for accountability.
4. Integration with existing systems:
- Develop APIs and connectors to seamlessly integrate data capture solutions with existing systems, such as CRM, ERP, or BI platforms, facilitating data exchange and interoperability.
- Customizable solutions we develop can be tailored to fit clients’ existing systems’ specific requirements and workflows, minimizing disruption and maximizing efficiency. In addition, we implement real-time or batch data synchronization mechanisms between data capture systems and existing databases or repositories, ensuring data consistency and accessibility across the organization.
Conclusion
Data capture technology keeps advancing along with the latest developments in AI and ML. Therefore, companies that still use manual data entry methods should switch to automated options to remain competitive. By adopting effective data capture strategies, businesses will be able to:
- Make decisions based on reliable insights and analytics
- Reduce manual effort, errors, and data processing time
- Personalize customer interactions, anticipate customer needs, and provide more responsive support
- Comply with data protection regulations by accurately collecting, storing, and managing sensitive information
- Track trends and identify opportunities to obtain a competitive edge in their industries
Lightpoint team develops custom solutions from scratch that allow businesses to collect the data they need and ensure data integrity and quality without sacrificing efficiency. Using state-of-the-art techniques and specialized know-how, we are at your disposal to build innovative solutions to meet your data capture needs. Contact us to conceptualize your project!