The Power of NLP in Content Marketing: Boosting Engagement and SEO

9 Apr 2024
Igor Kelly
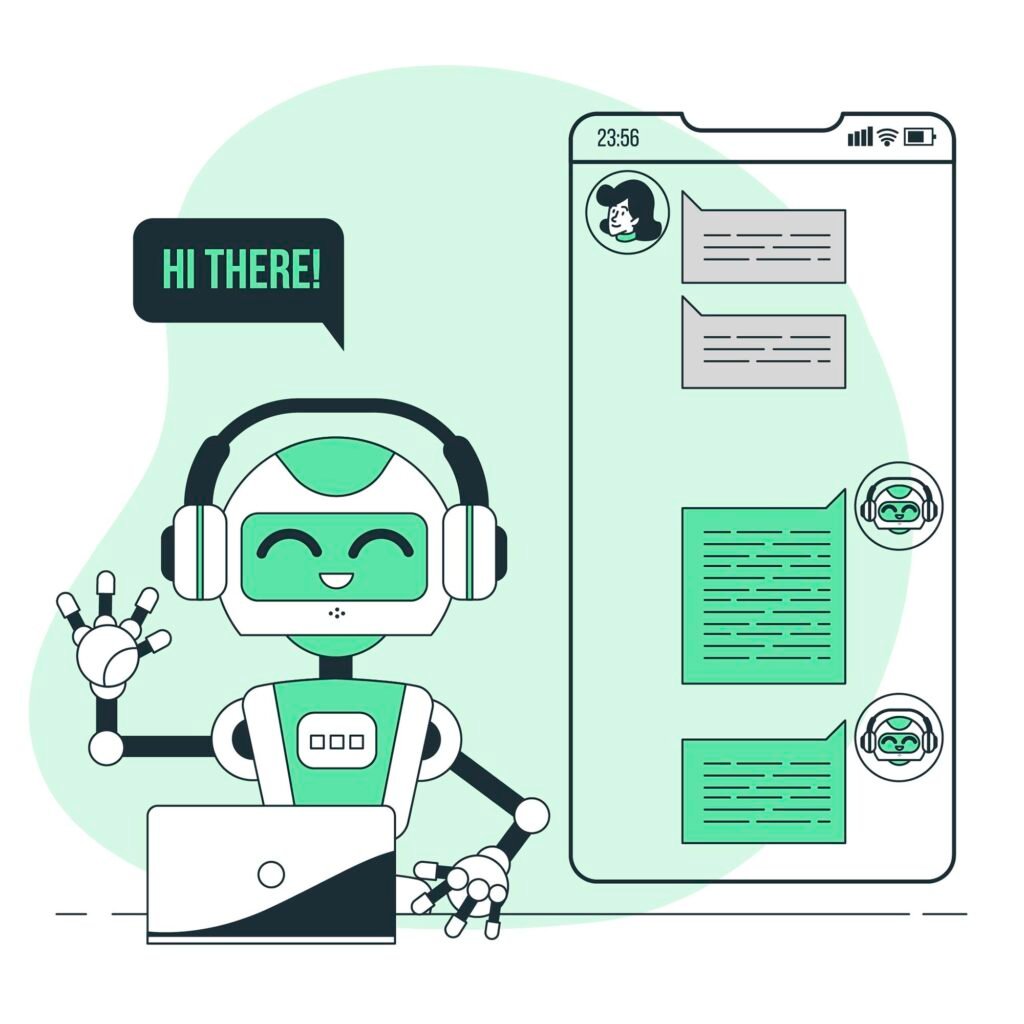
Natural language processing in marketing (NLP) bridges human language and machine understanding. NLP marketing meaning comprises the ability of computers to interpret texts and spoken words like humans do – with all their hidden nuances and underlying meanings – to improve marketing strategies. This means that machines can not only recognize and understand language but also react to it in a way that was previously only possible in interpersonal communication.
Therefore, more and more marketers are learning how to use NLP for marketing to analyze consumer behavior and language patterns.
Learn more about what is NLP in marketing, how it helps businesses gather more in-depth analytics about customers, and how businesses achieve SEO enhancement through NLP practices.
Understanding NLP: Key Concepts and Techniques
NLP in marketing uses machine learning to statistically analyze human-generated content and learn how to interpret it. During training, NLP models are fed examples of words and sentences in context and their interpretations. For example, an NLP model cannot understand that the word «orange» refers to the color and not the fruit. But after being shown thousands of examples – sentences like «I ate an orange» or «This car comes in orange» – the model can begin to understand the word and correctly interpret the difference between the meanings.
Given the complexity and inconsistency of human language, NLP is often based on deep learning, a more potent form of machine learning. Deep learning models can process unlabeled raw data but require large amounts of data to train. Deep learning also requires high computing power.
How does NLP work? NLP systems employ ML algorithms to parse vast unstructured data. The algorithms are trained to recognize patterns and draw conclusions based on those patterns. This is how it works:
- The user needs to enter a sentence into the NLP system.
- The NLP system then breaks the sentence into smaller parts of words, called tokens, and converts audio into text.
- The machine processes text data and generates an audio file accordingly, responding with synthesized audio derived from the processed text.
NLP marketing techniques can be roughly divided into two areas – NLP marketing methods and NLP in SEO. Here’s how they help enhance readers’ emotional resonance:
- NLP in marketing: NLP in marketing enables the creation of emotionally compelling content by analyzing language patterns and emotional cues in customer interactions. By leveraging behavioral targeting techniques, marketers can craft more impactful messaging that resonates with their audience, leading to increased engagement, brand affinity, and, ultimately, higher conversion rates.
- NLP in SEO: NLP optimization in SEO helps optimize content for emotional resonance by analyzing search queries, user intent, and content sentiment. By incorporating emotionally relevant keywords and phrases into website content, meta tags, and titles, SEO efforts can attract more targeted traffic and improve user engagement metrics such as click-through rates and time spent on a page, ultimately boosting search engine rankings and visibility.
Various NLP tasks break down human text and voice data in a way that helps the computer make sense of what it is receiving. Some of these tasks are listed below:
- Speech recognition (speech-to-text) reliably converts speech data into text data. Speech recognition is required for any application, such as following voice commands or answering spoken questions. What makes speech recognition particularly difficult is how people speak: quickly, with different stress and intonation, in various accents, and often with incorrect grammar.
- Grammatical tagging is determining the part of speech of a particular word or text based on its usage and context. For example, the part of speech identifies «make» as a verb in «I can make a paper plane» and as a noun in «What make of car do you own?»
- Disambiguation of word meaning is the selection of the meaning of a word with multiple meanings through a process of semantic analysis that determines the word that makes the most sense in the given context. Disambiguating the meaning of the word helps, for example, to distinguish the meaning of the verb «make» from «make the grade» and «make a bet.»
- NER (Named Entity Recognition) in marketing identifies words or phrases as valuable entities. NEM recognizes «Kentucky» as a city or «Fred» as a male first name.
- Coreference resolution involves determining whether and when two words refer to the same entity. The most common example is identifying the person or object referred to by a particular pronoun (e.g., «she» = «Mary»). Still, it can also involve identifying a metaphor or figure of speech in the text (e.g., a case in which a «bear» is not an animal but a large, muscular man).
- Sentiment analysis attempts to extract subjective qualities – attitudes, emotions, sarcasm, confusion, distrust – from the text. Semantic analysis in content marketing recognizes human feelings and opinions in the text, thus helping businesses improve products, services, and user experiences based on that understanding.
- Natural language generation is sometimes described as the opposite of speech recognition or speech-to-text; it is the task in which structured information is translated into human language.
Various NLP software for marketers helps with content creation and analysis. These tools provide insight into what the target group thinks about topics related to a company’s industry or brand. Below are some examples of these instruments:
- Google Natural Language API: This Google software analyzes texts utilizing entity recognition, syntactic analysis, and sentiment analysis. This allows the search engine to better understand the text’s deeper meaning, context, and structure.
- MarketMuse: Search engines use MarketMuse to research content using NLP and AI. They also analyze the quality of the content, and the tool helps develop content strategies. Contextual advertising with NLP improves the quality of content and increases the likelihood that the target audience will visit articles.
- SEMrush Writing Assistant: This tool is all about SEO optimization. Search engines base their suggestions on the articles with a specific keyword that performs best in Google search results. They also consider tone of voice or readability.
- Clearscope: Clearscope is a helpful writing tool. It improves the language understanding of language in marketing and increases the relevance of the content. For this purpose, the tool carries out keyword and competition analysis.
- GPT-3 by OpenAI: This content optimization platform uses NLP to help you write higher-rated, more relevant content. It offers detailed keyword and competition analysis.
- Amazon Comprehend: This NLP service from Amazon requires no knowledge of machine learning and is designed to help companies gather insights from emails, customer reviews, SocialExtract media, support tickets, and other text documents. The Amazon service uses sentiment analysis, part-of-speech extraction, and tokenization to understand the meaning behind the words.
- IBM Watson Tone Analyzer: This cloud-based solution from IBM is for social listening, chatbot integration, and customer service monitoring. The Tone Analyzer can detect customer emotions in postings, support calls, or chat conversations.
NLP Analytics for Marketing Performance
Investing time in content optimization with NLP can help you improve user experience, increase your traffic, and convert more leads. You can also develop a positive brand/employer reputation that ensures employee loyalty and increases customer loyalty.
- For employees, this means a reduction in fluctuation.
- For customers, this means increasing customer lifetime value (CLV).
The result could be increased repeat purchases, referrals, and potential upselling opportunities. NLP in marketing helps marketers put themselves in the mindset of their users (employees, customers) and look at things from their perspective. User experience improvement with NLP can raise the following guiding questions:
- Is the layout easy to understand?
- Is the text clear and concise?
- Is there too much information on one page?
- Does the design look appealing?
- Is it optimized for accessibility?
- Does the site load quickly?
- Is it easy to find what is needed?
- How visible are the buttons?
- Is there a navigation bar to help me find the right areas?
- Do I understand what I should do?
- Does the software work correctly?
- Does it make my work easier or more difficult?
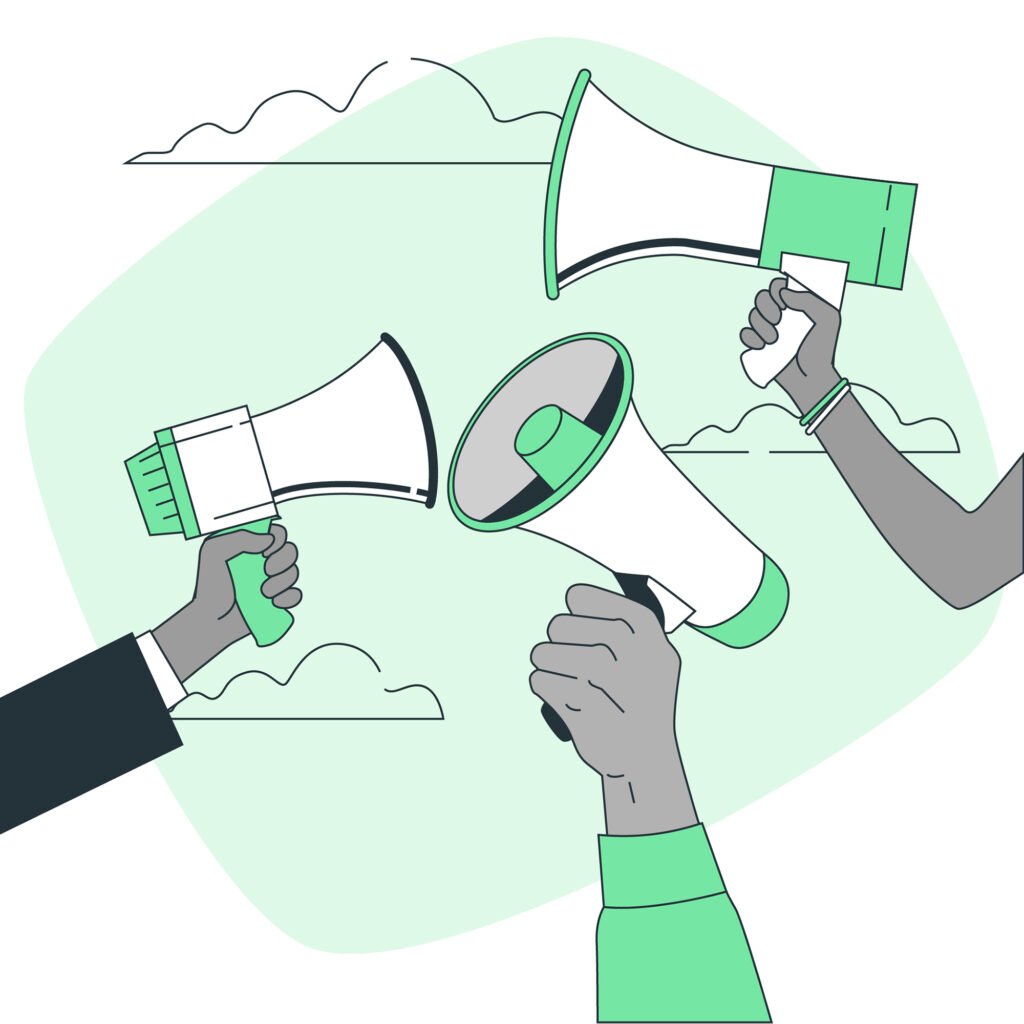
Various ways exist to recognize emotional resonance in NLP marketing and thus improve content’s relevance, understandability, or discoverability, as these questions break the text down into smaller components that can be analyzed more thoroughly. These are some tactics to improve AI-powered content strategies:
- Word segmentation: This task divides sentences into individual words that can be analyzed as units of meaning. Written words are separated by spaces in many languages, making word segmentation relatively easy. However, in some languages, such as Chinese, spaces do not separate words, and the process is more complicated.
- Disambiguation of the meaning of words: Many words have different meanings depending on how they are used in a sentence. Although it may be easy for you to tell the difference between «he has good taste» and «the soup tastes good,» a computer must understand all possible word meanings and which one makes the most sense in a given sentence.
- Part-of-speech tagging: Each word in a text selection is tagged as a part of speech, such as a noun, verb, or adjective. This helps the NLP program understand the relationship of a word to the words around it and the meaning of the overall text.
- Dependency parsing: This technique analyzes sentences to determine the relationships between parts of the sentence and helps the program understand the grammar and meaning of the sentence.
At Lightpoint, we rely on industry-proven practices as we provide natural language processing as a service. Here are a few principles we adhere to when implementing NLP-driven engagement strategies:
1. Augment content recommendations:
- Analyze user interactions with the website to understand their preferences and interests.
- Utilize NLP algorithms to recommend relevant content, such as articles, products, or videos, based on their browsing history, search queries, and contextual information.
2. Generate dynamic content:
- Employ NLP to dynamically analyze user demographics, behavior, and real-time data to generate personalized content. This can include personalized product recommendations, targeted promotions, or tailored messages that resonate with the user’s interests and preferences.
3. Integrate sentiment analysis and feedback:
- Implement sentiment analysis using NLP to understand user sentiment towards content or products.
- Integrate feedback mechanisms to gather user opinions and sentiments, allowing for continuous improvement and personalized adjustments to content or offerings based on user feedback.
Such a robust approach to conversational marketing with NLP ensures true accessibility. Also, it lowers the inhibition threshold for less technically savvy company employees to use analytics in their daily work.
NLP’s Impact on SEO
NLP-powered sentiment analysis is a smaller subset of social listening or social media monitoring. While social listening refers to conversations that people are having around your brand in general, sentiment analysis is about identifying opinions and then determining whether the person who posted comments has a negative or positive one or represents a neutral opinion about your company. Thus, updating content relevance with NLP can deliver valuable data from business reviews.
If a post or review has a lot of positive connotations like «brilliant,» «awesome,» or «amazing,» then the tool will exclude the general sentiment as positive.
While social listening refers to listening in on conversations that people are having around your brand in general, sentiment analysis is about identifying opinions and then determining whether the person who posted comments has a negative, positive one or represents a neutral opinion about your company.
Here are two examples of comments that could be analyzed using sentiment analysis:
- «Arrr, you pirates and buccaneers! I recently tried this serum on my face, and I’m really impressed! This stuff made me shine like a treasure chest full of jewels!»
- «I’ve been using the product for several years, but after the dispenser changed, the concentrate was no longer thick but diluted to an almost water-based consistency. Therefore I think the reduction was drastic and way too much. You really want to know why you should spend so much money on it?! In any case, I won’t let myself be taken for a ride.»
In the first comment, the sentiment is clearly positive, expressing satisfaction and recommendation. In contrast, the second comment conveys a negative sentiment, indicating dissatisfaction and frustration with the company’s packaging solution.
Although natural language processing can’t do your job for you, it can also help you better listen to your customers – literally. NLP aids in optimizing content for voice search by analyzing conversational language patterns and understanding user intent. By translating spoken words into text, voice command recognition software recognizes the meaning behind the words, categorizes the user’s intent, and generates appropriate responses. Here’s how it works:
- Voice input: The user speaks a command or query into the voice search system.
- Speech recognition: NLP software converts spoken words into text, employing algorithms to accurately transcribe the audio input into a textual format.
- Natural Language Understanding (NLU): The system comprehends the meaning behind the transcribed text, analyzing syntax and semantics to identify intents, entities, and context within the user’s input.
- Intent classification: Based on the understanding derived from NLU, the system categorizes the user’s input into specific intents or actions, determining what the user is trying to accomplish.
- Response generation: The system generates an appropriate response or takes the necessary action based on the identified intent, providing relevant information or executing commands as needed.
Continuously learning from user interactions, the NLP software refines its language models and algorithms to enhance accuracy and adaptability over time. NLP-driven voice search optimization helps to create more effective and personalized responses in future user interactions.
Successful Implementation of NLP in Content Marketing
Natural language processing in marketing is the driving force behind machine intelligence in many modern everyday applications. The following examples prove this:
- Spam detection: When you think of spam detection, you might not think of an NLP solution, but the best spam detection technologies leverage NLP’s text classification capabilities to scan emails for elements of language that often indicate spam or phishing. These indicators include, but are not limited to, excessive use of financial terms, typically poor grammar, threats, undue urgency, misspelled company names, and more. Spam detection is one of the few NLP problems that experts consider «largely solved» (although you might argue that this doesn’t reflect your personal experience with email).
- Machine translation: Truly useful machine translation involves more than replacing words in one language with words in another. A good translation must accurately capture the meaning and tone of the source language and translate it into a text with the same meaning and desired effect in the target language. Machine translation programs are making significant progress in terms of accuracy. A good way to test a machine translation program is to translate a text into another target language and then back into the source language.
- Virtual agents and chatbots: Virtual agents use voice recognition to recognize patterns in voice commands and natural language generation to respond with appropriate actions or helpful comments. Chatbots enable similarly good results in response to typed text input. The best of them also learn to recognize contextual clues in human queries and use these to offer even more appropriate answers or options over time. The next improvement for these applications is question answering, that is, the ability to respond to our questions – whether expected or not – with relevant and helpful answers in their own words.
- Social media sentiment analysis: NLP has become an essential business tool for unlocking hidden data from social media channels. Sentiment analysis can analyze the language used in social media posts, replies, reviews, etc., to determine attitudes and emotions in response to products, promotions, and events – information companies can use for product designs, advertising campaigns, and more.
- Text summarization: Natural language processing in marketing processes large amounts of digital text and produces summaries and synopses for indexes, research databases, or busy readers who do not have time to read the entire text. The best text summarization applications use semantic inference and natural language generation for marketing tasks, such as adding useful context and inferences to summaries.
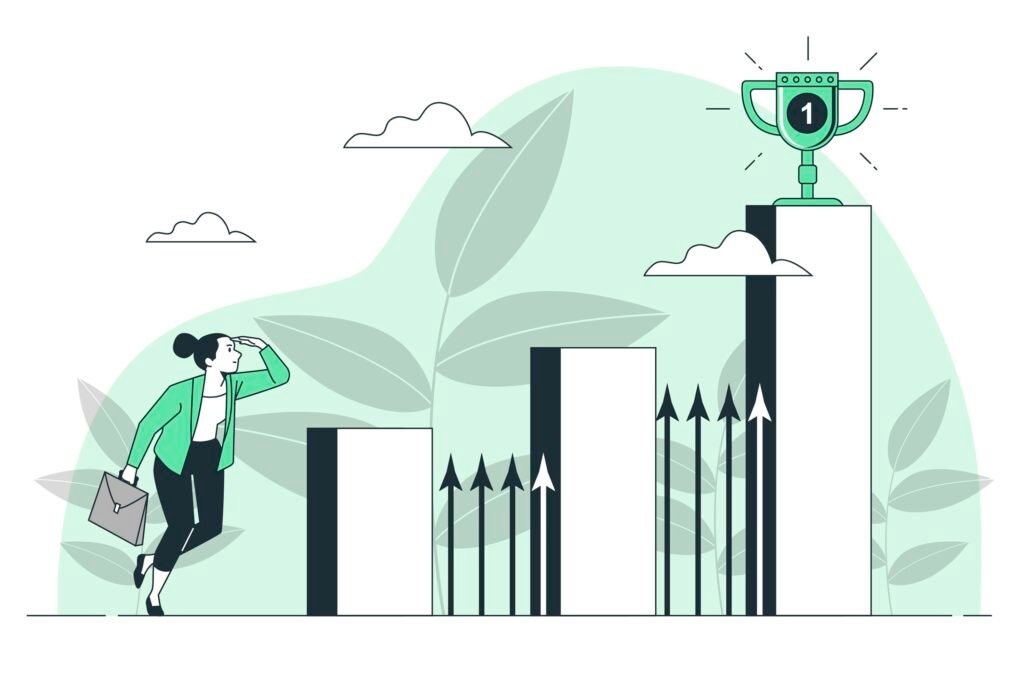
The possible applications for natural language processing in the digital world are endless, and new ones are being added daily as more companies and industries recognize its benefits. Difficult issues still need to be clarified by humans, but smaller tasks can already be automated using NLP systems. With increasing technical progress, increasingly complex applications can be expected in the future, and artificial intelligence software development services will help solve problems that were previously only science fiction.
Conclusion
The evolutionary development of Natural Language Processing sets new standards in the field of content creation. Thoughts, research, opinions, facts, and feedback flow into the digital world via social media feeds, court records, electronic medical records, contact center records, warranty claims, etc. NLP in SEO unlocks insights hidden in the streams of words. With NLP capabilities, businesses can automate various tasks, such as:
- Process, analyze, and archive large documents
- Analyze customer feedback or call center records to derive actionable insights from call analytics
- Run chatbots for automated customer service Personalization in marketing content
- Classify and extract text
NLP solutions do not have to be extremely complex or extremely expensive. Lightpoint helps companies do things that make a difference for them, and our expertise allows us to create elegant, scalable, and effective solutions.
Contact us and let us develop a revenue-boosting NLP solution for your business!